November 2022
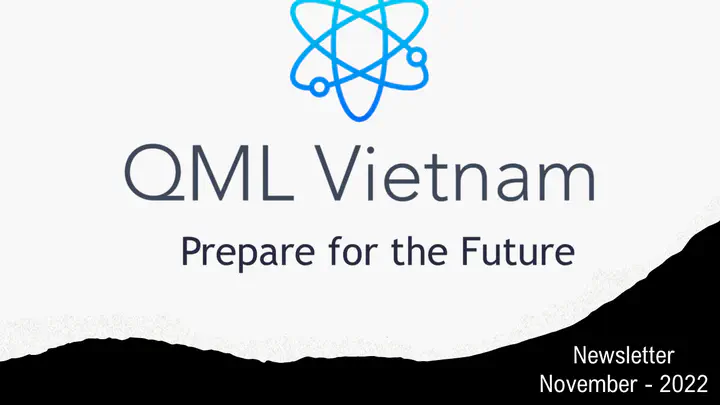
News π°
-
D-Wave Says Revenue Up, Added More Commercial Customers in Q3
-
Unileverβs Head of R&D Alberto Prado Talks Quantum at IoT World & The AI Summit Austin
Hackathon and Internship π
Publications π
Reservoir Computing via Quantum Recurrent Neural Networks
Recent developments in quantum computing and machine learning have propelled the interdisciplinary study of quantum machine learning. Sequential modeling is an important task with high scientific and commercial value. Existing VQC or QNN-based methods require significant computational resources to perform the gradient-based optimization of a larger number of quantum circuit parameters. The major drawback is that such quantum gradient calculation requires a large amount of circuit evaluation, posing challenges in current near-term quantum hardware and simulation software. In this work, we approach sequential modeling by applying a reservoir computing (RC) framework to quantum recurrent neural networks (QRNN-RC) that are based on classical RNN, LSTM and GRU. The main idea to this RC approach is that the QRNN with randomly initialized weights is treated as a dynamical system and only the final classical linear layer is trained. Our numerical simulations show that the QRNN-RC can reach results comparable to fully trained QRNN models for several function approximation and time series prediction tasks. Since the QRNN training complexity is significantly reduced, the proposed model trains notably faster. In this work we also compare to corresponding classical RNN-based RC implementations and show that the quantum version learns faster by requiring fewer training epochs in most cases. Our results demonstrate a new possibility to utilize quantum neural network for sequential modeling with greater quantum hardware efficiency, an important design consideration for noisy intermediate-scale quantum (NISQ) computers.Abstract:
Benchmarking Adversarially Robust Quantum Machine Learning at Scale
Machine learning (ML) methods such as artificial neural networks are rapidly becoming ubiquitous in modern science, technology and industry. Despite their accuracy and sophistication, neural networks can be easily fooled by carefully designed malicious inputs known as adversarial attacks. While such vulnerabilities remain a serious challenge for classical neural networks, the extent of their existence is not fully understood in the quantum ML setting. In this work, we benchmark the robustness of quantum ML networks, such as quantum variational classifiers (QVC), at scale by performing rigorous training for both simple and complex image datasets and through a variety of high-end adversarial attacks. Our results show that QVCs offer a notably enhanced robustness against classical adversarial attacks by learning features which are not detected by the classical neural networks, indicating a possible quantum advantage for ML tasks. Contrarily, and remarkably, the converse is not true, with attacks on quantum networks also capable of deceiving classical neural networks. By combining quantum and classical network outcomes, we propose a novel adversarial attack detection technology. Traditionally quantum advantage in ML systems has been sought through increased accuracy or algorithmic speed-up, but our work has revealed the potential for a new kind of quantum advantage through superior robustness of ML models, whose practical realisation will address serious security concerns and reliability issues of ML algorithms employed in a myriad of applications including autonomous vehicles, cybersecurity, and surveillance robotic systems.Abstract:
Quantum Feature Maps for Graph Machine Learning on a Neutral Atom Quantum Processor
Using a quantum processor to embed and process classical data enables the generation of correlations between variables that are inefficient to represent through classical computation. A fundamental question is whether these correlations could be harnessed to enhance learning performances on real datasets. Here, we report the use of a neutral atom quantum processor comprising up to 32 qubits to implement machine learning tasks on graph-structured data. To that end, we introduce a quantum feature map to encode the information about graphs in the parameters of a tunable Hamiltonian acting on an array of qubits. Using this tool, we first show that interactions in the quantum system can be used to distinguish non-isomorphic graphs that are locally equivalent. We then realize a toxicity screening experiment, consisting of a binary classification protocol on a biochemistry dataset comprising 286 molecules of sizes ranging from 2 to 32 nodes, and obtain results which are comparable to those using the best classical kernels. Using techniques to compare the geometry of the feature spaces associated with kernel methods, we then show evidence that the quantum feature map perceives data in an original way, which is hard to replicate using classical kernels.Abstract:
Symmetric Tensor Networks for Generative Modeling and Constrained Combinatorial Optimization
Constrained combinatorial optimization problems abound in industry, from portfolio optimization to logistics. One of the major roadblocks in solving these problems is the presence of non-trivial hard constraints which limit the valid search space. In some heuristic solvers, these are typically addressed by introducing certain Lagrange multipliers in the cost function, by relaxing them in some way, or worse yet, by generating many samples and only keeping valid ones, which leads to very expensive and inefficient searches. In this work, we encode arbitrary integer-valued equality constraints of the form Ax=b, directly into U(1) symmetric tensor networks (TNs) and leverage their applicability as quantum-inspired generative models to assist in the search of solutions to combinatorial optimization problems. This allows us to exploit the generalization capabilities of TN generative models while constraining them so that they only output valid samples. Our constrained TN generative model efficiently captures the constraints by reducing number of parameters and computational costs. We find that at tasks with constraints given by arbitrary equalities, symmetric Matrix Product States outperform their standard unconstrained counterparts at finding novel and better solutions to combinatorial optimization problems.Abstract:
Quantum machine learning for chemistry and physics
Machine learning (ML) has emerged as a formidable force for identifying hidden but pertinent patterns within a given data set with the objective of subsequent generation of automated predictive behavior. In recent years, it is safe to conclude that ML and its close cousin, deep learning (DL), have ushered in unprecedented developments in all areas of physical sciences, especially chemistry. Not only classical variants of ML, even those trainable on near-term quantum hardwares have been developed with promising outcomes. Such algorithms have revolutionized materials design and performance of photovoltaics, electronic structure calculations of ground and excited states of correlated matter, computation of force-fields and potential energy surfaces informing chemical reaction dynamics, reactivity inspired rational strategies of drug designing and even classification of phases of matter with accurate identification of emergent criticality. In this review we shall explicate a subset of such topics and delineate the contributions made by both classical and quantum computing enhanced machine learning algorithms over the past few years. We shall not only present a brief overview of the well-known techniques but also highlight their learning strategies using statistical physical insight. The objective of the review is not only to foster exposition of the aforesaid techniques but also to empower and promote cross-pollination among future research in all areas of chemistry which can benefit from ML and in turn can potentially accelerate the growth of such algorithms.Abstract: